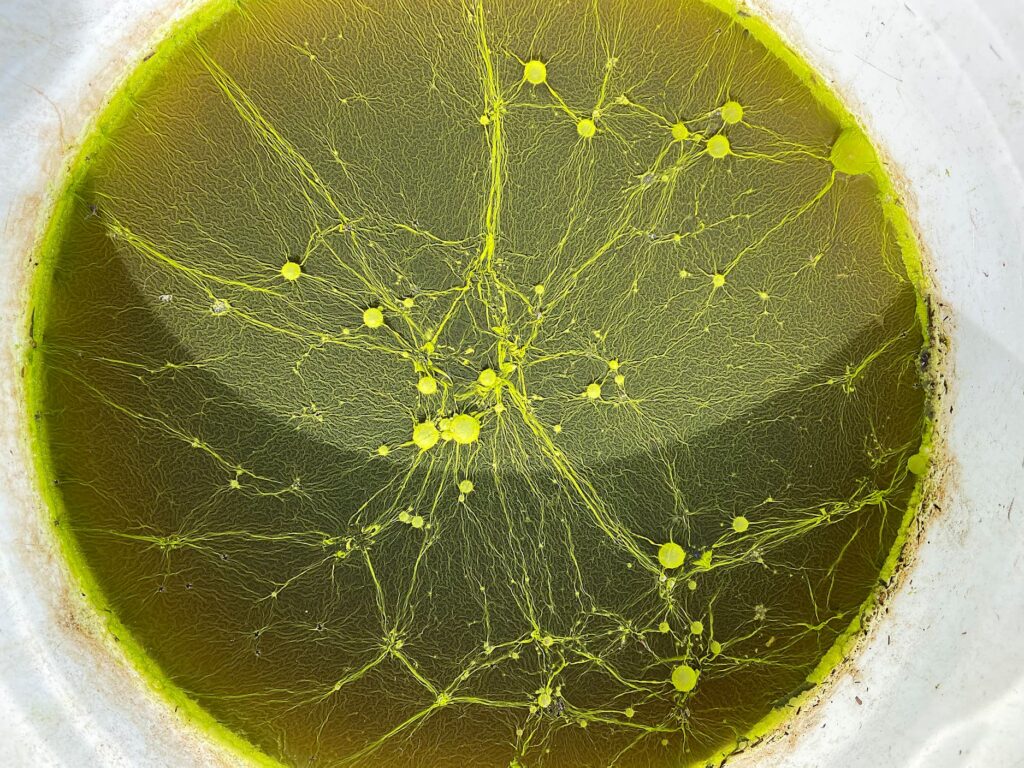
The systematic methods that investigators use when collecting evidence vary wildly; they depend both on the field that’s being studied as well as the discipline the investigator applies to the investigation. But I think there are some best practices that can be pieced together to guide any investigator who wishes to follow a scientific and rigorous process. At least – I think there’s a basic starting point. What I’m proposing here are fundamentals that might be used by anyone – scientists, investigators, citizens, citizen-scientists – in the study of anything – from common physical events to rare mysteries. Why are all the frogs in this pond dead? Why did Chernobyl melt down? Why won’t my car start? Does Bigfoot exist? All good questions like these should be approached by the scientific method of collecting and logically assembling evidence, and that method will only work if strategized properly. And since evidence is such a foundation of these methods, I’ll try to highlight the different types of evidence in bold text throughout.
First, a disclaimer about my credibility as a source for this: I’m not Sherlock Holmes, nor do I have much experience with investigations outside the realm of industrial system equipment failures, some toxicology studies, and data analytics. My knowledge of evidential probability comes from books, some involvement in legal claims, and – to some extent – from my experiences teaching workshops in decision-making and risk assessment. I’m sure there are detectives, journalists, law clerks, machinists, biologists, and a million other types of people whose experience makes their ideas better than mine. (The only thing I’ve got over them is the fact that mine are published in a place that you – the reader – happened to have found!)
Investigators, researchers, process specialists, et. al. share a keen interest in defining some root event. In scientific research it’s more about forming a hypothesis of a cause-effect relationship, and in business process analysis it’s about simply defining the “problem” that’s under consideration. Here I’ll talk about it as a Fact In-Issue. However we want to define its endpoint, this step is the grounding for an inferential network, which gives a framework for the gathering of evidence. The network is a series of paths connecting nodes of evidence. And it’s a living model, in that we can build upon and modify it with different legs during discovery. The important part is that the path leads to a single destination – a simple yes or no hypothesis. This is the best way to give the research the focus it needs for efficiency. I mean, you can’t research everything, right? You need to prioritize what you collect, somehow. And, as you collect evidence, you should be assessing its strength relative to something – to the linkage it has to its neighbor on the network. An inferential connection can be circumstantial instead of direct, in which case we might want to look for some direct or corroborative evidence. If a node seems to be supported by strong demonstrative evidence, such as a map by the US Archeological Survey, then no further collecting may be necessary. But weaker evidence such as hearsay testimonial evidence may deserve ancillary evidence to bear upon its credibility. So that means – while you’re at it surveying or visiting a site or plundering a library – it might be a good time to expand the scope of what you’re collecting.
The assignment of a single Fact In-Issue also ensures some rigor around the research. That is, it gives a singular purpose to the investigation, keeping it from being an exercise in hoarding answers in the absence of a question (a post hoc analysis, which in some cases is a fallacy). Again, this goes back to the efficiency of the exercise and making best use of the investigator’s time and the research funding. And the merger of all those possibilities into a single point helps to paint another tactical picture for the researcher – it shows the “white spaces” between any brainstormed chain of evidence. That is, the good investigator must also look for dissonant evidence (that either conflicts or contradicts with the assumptions) as well as the body of evidence – either positive or negative – that might be missing. A network model also makes obvious the wasted effort of gathering too much evidence that corroborates (and possibly dangerously reinforces) the same premise. We might think of this inferential network model as a sort of cork board that’s used in detective movies (and maybe by real-life detectives). Facts and photos and locations are collected in one place, pinned on a board for the detectives to stare at, appreciating the new leads and trying to figure out what’s missing. (Photo below is a Wigmore diagram from the excellent book: The Evidential Foundations of Probabilistic Reasoning by David Schum)
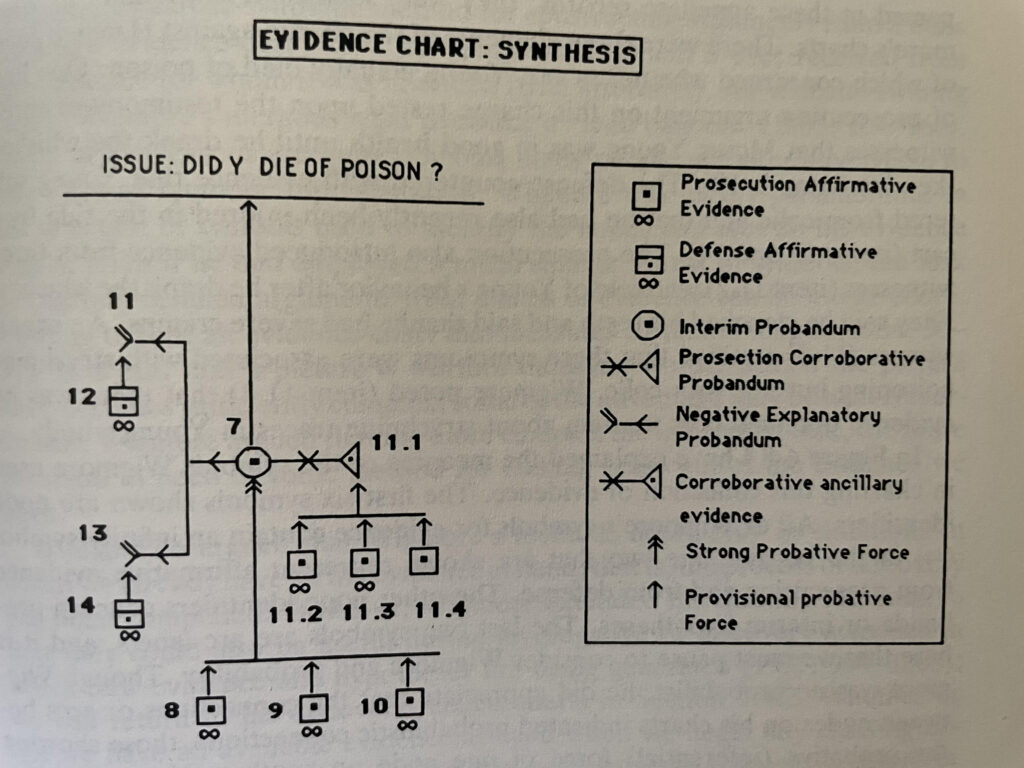
So now we have a model, and the scientific approach is to try to dress up that model with evidence. When we go shopping for those dressings, it’s important to look in all the right places. And that’s when we need a categorical template to focus our research. Maybe the best MECE set of categories comes from Root Cause Analysis (RCA), in which the evidence is explored against three categories: human skill, physical factors, and organizational influences. Those categories are further subdivided depending on the type of system that’s experienced the problem. If you’re talking about machines, the human skill factor is usually only about “operator error,” as in whether the input code was fat-fingered at the control desk or whether the controller wasn’t really qualified to be sitting at that desk! And the organizational influences are often just a subset of the operator’s world of operating procedures and sick time policies and such. Some of these factors can be discovered through a Barrier Analysis – an approach that considers all of the procedural, system, and policy mechanisms that were in place to prevent the failure. When interviewing operators about root cause of an event, it’s useful to include a question or two about the safety barriers, input screen hard errors, quality checks, and other preventative controls that they normally encounter when doing their job. Often a failure can be traced to a faulty barrier, and we might also learn that failures happen because there are too many useless, distracting barriers in place!
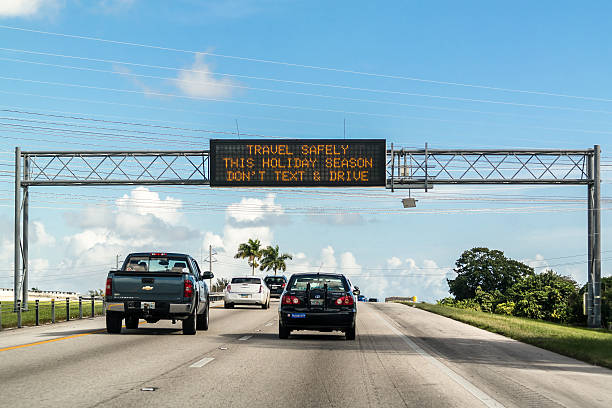
Another subcategory of detail to consider is the way the RCA of physical systems is very focused on segments of the design-build lifecycle, from possible faults in design through material selection, fabrication, maintenance, and the general process controls like configuration management and verification. That sort of breakdown should be entertained if there’s any physical system involved in the Fact In-Issue. And when it’s about life instead of machines, such as in medical diagnostics, the environmental factors that have surrounded the patient may be worthy of much more interest and more evidence. And in the study of problems in social sciences, such as the political upheaval in a nation or the origins of a psychiatric disorder, the organizational influences category should be expanded to cover cultural influences, language, laws, media, etc.
Each piece of evidence should be examined for its credibility to some extent upon collection. Again, the researcher deserves to know whether she’s wasting her time – going down a rabbit hole, chasing a red herring, or such. And that means being able to look ahead and give some thought to what’s being collected. The star-watchers on astronomical observatory teams need to know enough about theory and enough about telescopes to be able to focus their attention (and sleep schedules) around short watching periods. The credibility of tangible evidence is indeed subject to the sensitivity or distortion of the measuring device and the receiver of the signal. Really the best way to consider that strength is by accessing more data – ancillary evidence used to assess the probability of family-wise errors in the signal detection. Yes, the enemy aircraft alarm went off, but what was the likelihood of false alarms? And what was the likelihood that there actually was an enemy aircraft in the air? If the first is ten percent and the latter is only half a percent, no rational person should conclude that it’s 90% likely that an enemy aircraft was there at the time! Well, all of this is just about signals – tangible evidence that is actual artifact should have its credibility judged by its chain of custody, from provenance through all handling. And all of these qualities about tangible evidence should be used to tactically pivot your research plan to collect more … or go look someplace else!
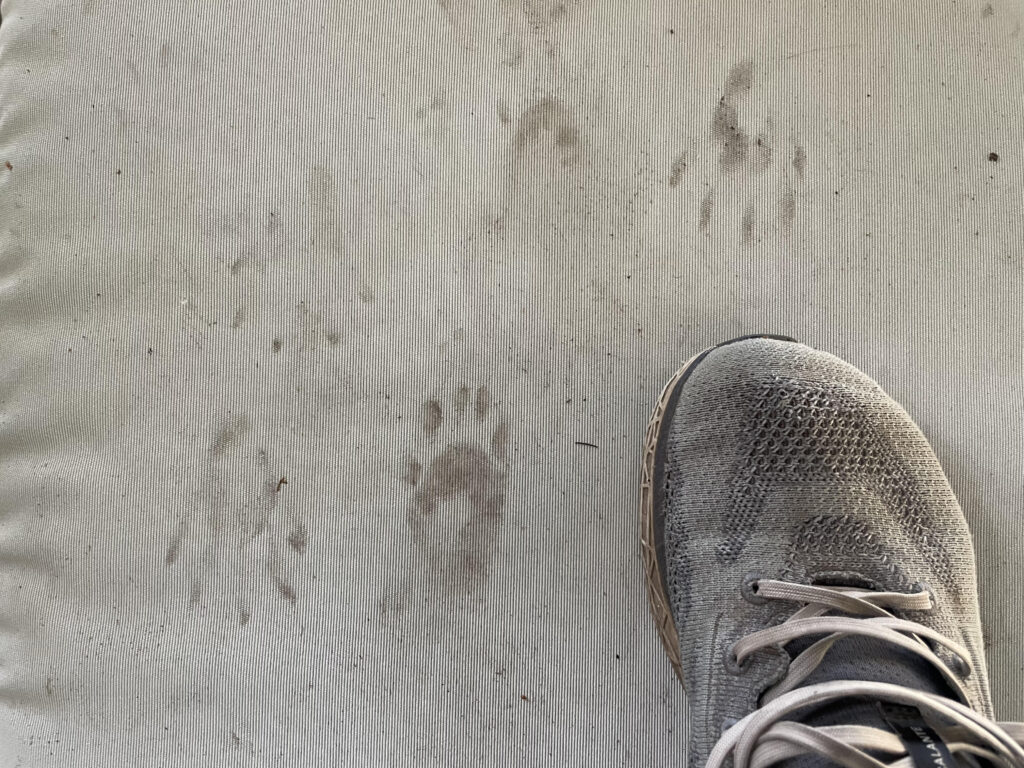
Arguably most deserving of the researcher’s attention (and subsequent tactics) is the quality of testimonial evidence. A witness testimony should be judged for its justified true belief, or whether it rests on non-defective evidence. There are three spectra for this: the veracity (whether the witness is reporting what they actually believe happened), the objectivity (whether the witness belief corresponds to what they think they witnessed), and the Observational Sensitivity (whether the witness’ senses worked properly). And, as described earlier, the strength of testimonial evidence is often judged by whether it’s just hearsay, second-hand, versus having been directly witnessed. We also need to consider whether the witness is giving opinion evidence, testifying to some truth that’s really only a mental model, their own inferential connection between two different facts (or – even worse – a fact to a belief). If a court is collecting testimonial evidence from witnesses, these nuances are openly called out in objections and jurors are instructed to ignore it. A good researcher or citizen scientist should do the same.
When listening to or reading about testimonial evidence, the investigator should also try to be discerning about their own role. It’s all too easy to commit hermeneutical injustice, in which there’s a failure by the interrogator to fully understand the testimony. If you’re researching a phenomenon that occurred off the coast of Vietnam, for example, it might be useful to know some Vietnamese or to involve a native speaker in your research. You may after all be missing out on some very important evidence from the newspaper accounts of local fishermen or the subtle change of meaning in the tonal language used in any recorded account by local witnesses. Even more egregious – and even easier to avoid – is testimonial bias, in which the interrogator discounts or discredits the value of the witness testimony simply because the witness is from a different culture, has different beliefs, etc. The redneck drunkard may after all be speaking the truth about a sexual encounter with Bigfoot, but it’s far too easy and convenient to pooh-pooh the testimony as the rant of a some drunk redneck and quickly head back to town. Maybe you missed something important! A good investigator has that sense of humility that makes them a good listener, without judgment and with nothing but respect for the witness.
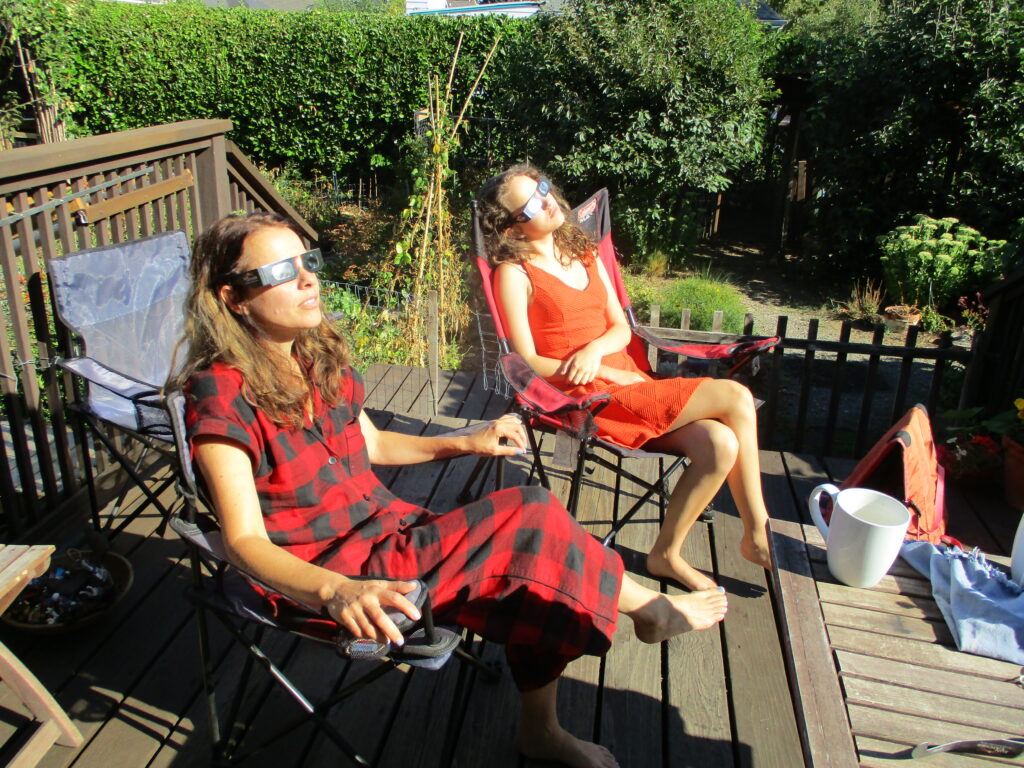
You are witnessing witnesses witnessing
I shouldn’t end without advocating for honesty in sampling. By “honesty” I mean being sure to get a representative sample for every population that you’re trying to assess. There are some excellent statistical methods for doing this, and those are best demonstrated in data science and most of the good medical research. There are also some cool shortcuts that are available through methods like rank order sampling, stratified sampling, and application of the Neyman-Pearson technique of using different p-values for each tail of the probability distribution (not to be confused with the crime of p-hacking). And above all, honesty is about discounting biases so that you’re able to connect with the entire population, not just taking what’s handy (availability bias) or what’s most likely to support your preconceived conclusion (data dredging, I think). This kind of honesty requires a bit of that humility I was talking about.
Well, I was hoping to keep this short and simple, but I’ve probably made a mess of it. Anyway, I hope you got a little something out of it. My thesis here is that evidence must be collected strategically, with an eye to getting the best value out of logical connections between every item. And in order to do that, the investigator has to take on some of the fundamental traits of any good scientist: logical reasoning, humility, and maybe a bit of project management planning.
by Mark Nadeau (2023)
This is one of what may be many articles that I’ll write on scientific analysis. Someday soon these will appear – in more polished form – in a book I’m writing. In the meantime, I appreciate any input and ideas that you want to share. I think you can comment on these pages, can’t you? Otherwise, consider sending a message to me at emkhos.development@gmail.com.
More Emkhos writing in The Library